Propensity score matching is a statistical technique used in observational studies to reduce bias and improve the validity of causal inference. It is a tool that has gained popularity in various fields, including medicine, education, and social sciences. In simple terms, propensity score matching is a method for creating comparable groups in non-experimental research by matching individuals with similar characteristics. As a kitchen sink model, propensity score matching has been widely used in evaluating the effectiveness of different kitchen sink models. With the increasing demand for evidence-based decision making, it has become essential for applied practitioners to understand the concept of propensity score matching and its applications in real-world settings. In this article, we will provide a conceptual review of propensity score matching and its relevance in kitchen sink model research.Propensity Score Matching: A Conceptual Review for Applied Practitioners
Observational studies are studies in which the researcher does not control the assignment of participants to treatment or control groups. Instead, the assignment is done by nature or by the participants themselves. This lack of random assignment may result in selection biases, making it challenging to establish a causal relationship between the treatment and outcome. This is where propensity score matching comes in. Propensity score matching works by creating a propensity score for each individual, which is the probability of being assigned to a particular treatment group based on their observed characteristics. These characteristics, or covariates, may include age, gender, socioeconomic status, and other relevant factors. Once the propensity scores are calculated, individuals with similar scores are matched, creating comparable treatment and control groups.Propensity Score Matching in Observational Studies
In educational research, propensity score matching has been used to evaluate the effectiveness of various educational interventions, such as school programs, teaching methods, and educational policies. By matching individuals with similar propensities, researchers can isolate the effects of the intervention from other confounding factors, providing more reliable evidence of its impact. One of the main advantages of propensity score matching in educational research is that it allows for the comparison of groups with different characteristics. For example, researchers can match students from different schools or with different demographic backgrounds, making it easier to generalize the findings to a larger population.Propensity Score Matching: A Primer for Educational Researchers
Program evaluation is an essential aspect of applied research, as it helps determine the impact of interventions and policies on the target population. Propensity score matching is a valuable tool for program evaluation, as it can control for multiple variables simultaneously, reducing the risk of confounding factors. This makes it an ideal technique for estimating the causal effects of complex interventions. Moreover, propensity score matching can be used in combination with other statistical techniques, such as regression analysis or difference-in-differences, to improve the robustness of the findings. This allows for a more comprehensive evaluation of the program's impact and can provide valuable insights for decision making.Propensity Score Matching: A Multivariate Technique for Program Evaluation
Given the increasing use of propensity score matching in various fields, a comprehensive review of its strengths and limitations is crucial for applied practitioners. While propensity score matching has been shown to reduce bias and improve the validity of causal inference, it is not a perfect technique and has its own set of limitations. One of the main limitations of propensity score matching is that it relies on the assumption of unconfoundedness, which states that all relevant variables have been included in the propensity score model. This assumption may not always hold, leading to biased results. Additionally, the quality of the matching depends on the quality of the covariates used, which may not always be readily available.Propensity Score Matching: A Comprehensive Review
Non-experimental research, also known as observational research, is common in many fields, including kitchen sink model research. In such studies, researchers do not have control over the assignment of individuals to treatment or control groups, making it challenging to establish causality. Propensity score matching offers a solution to this problem by creating comparable groups that can be used to estimate causal effects. Moreover, propensity score matching can also be used to assess the balance of covariates between treatment and control groups, providing additional evidence of the validity of the results. This makes it a valuable tool for causal inference in non-experimental research.Propensity Score Matching: A Method for Causal Inference in Non-Experimental Research
In addition to its applications in kitchen sink model research, propensity score matching has also been widely used in program evaluation and policy research. By creating comparable groups, researchers can determine the impact of various policies and interventions on different outcomes, such as health, education, and employment. This has important implications for decision making, as policymakers can use the findings from propensity score matching to inform the development of effective policies and programs that benefit the target population.Propensity Score Matching: A Tool for Program Evaluation and Policy Research
For researchers and practitioners looking to use propensity score matching in their studies, it is essential to understand the steps involved in the process. These include selecting relevant covariates, estimating the propensity scores, and matching individuals based on their scores. It is also crucial to assess the balance of covariates between treatment and control groups and to conduct sensitivity analyses to test the robustness of the results. Furthermore, it is essential to understand the limitations of propensity score matching and to use it in combination with other statistical techniques to improve the validity of the findings. By following these guidelines, researchers and practitioners can effectively use propensity score matching to answer important research questions and inform decision making.Propensity Score Matching: A Guide for Researchers and Practitioners
Finally, propensity score matching is a valuable method for estimating causal effects in observational studies. By creating comparable groups, researchers can isolate the effects of the treatment from other confounding factors, providing more reliable evidence of causality. Moreover, propensity score matching can be used to estimate not only the average treatment effect but also the effects for specific subgroups within the population. This makes it a powerful tool for understanding the differential impacts of interventions and policies, which is crucial for making informed decisions.Propensity Score Matching: A Method for Estimating Causal Effects in Observational Studies
In conclusion, propensity score matching is a valuable tool for applied practitioners in various fields, including kitchen sink model research. By creating comparable groups, propensity score matching can reduce bias and improve the validity of causal inference in non-experimental research. However, it is essential to understand its strengths and limitations and to follow best practices when using this technique. With the increasing demand for evidence-based decision making, propensity score matching is a method that all applied practitioners should have in their research toolkit.Propensity Score Matching: A Practical Guide for Researchers and Practitioners
A Comprehensive Guide to the Kitchen Sink Model Propensity Score
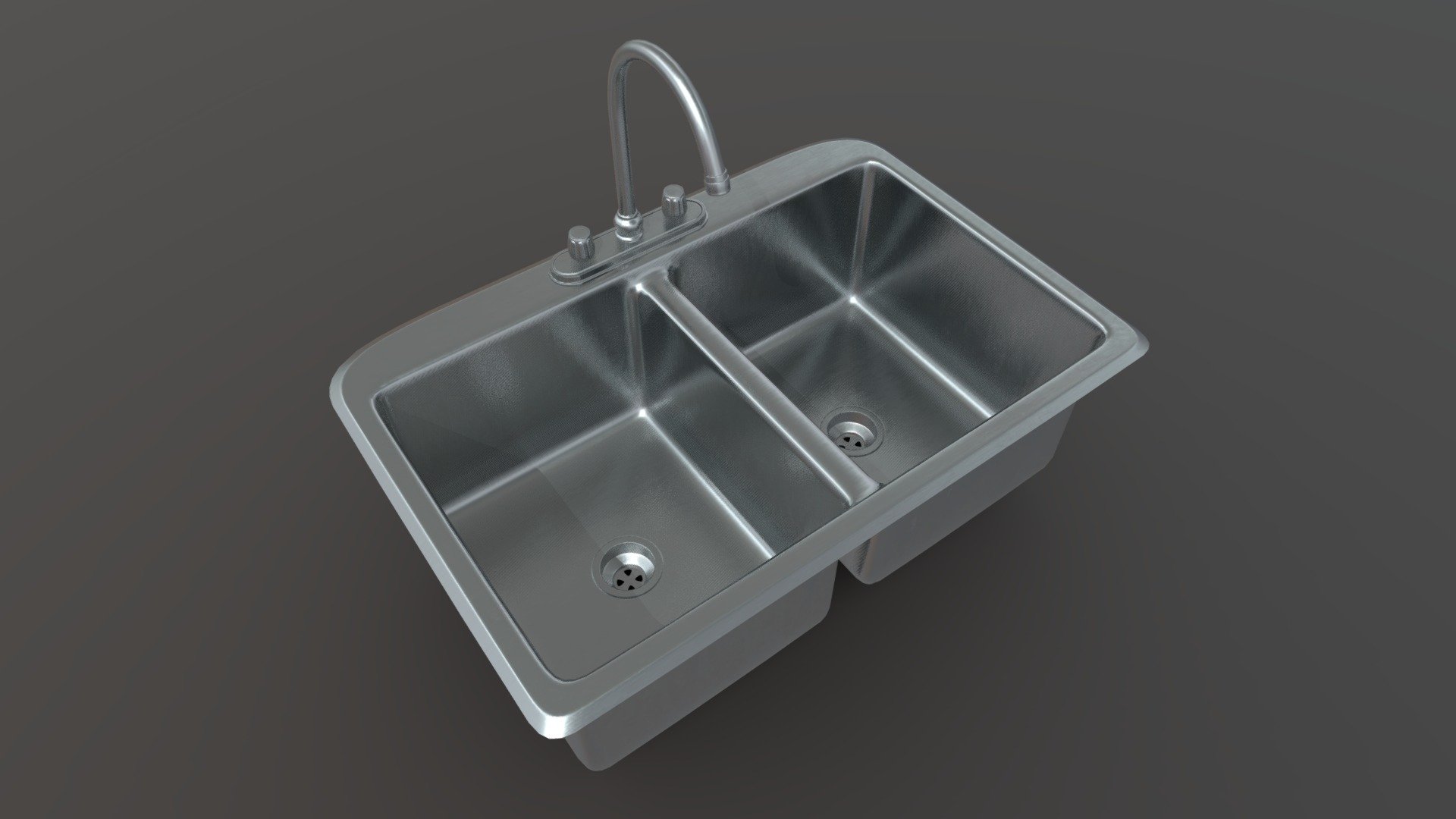
Understanding the Kitchen Sink Model

What is the Propensity Score?
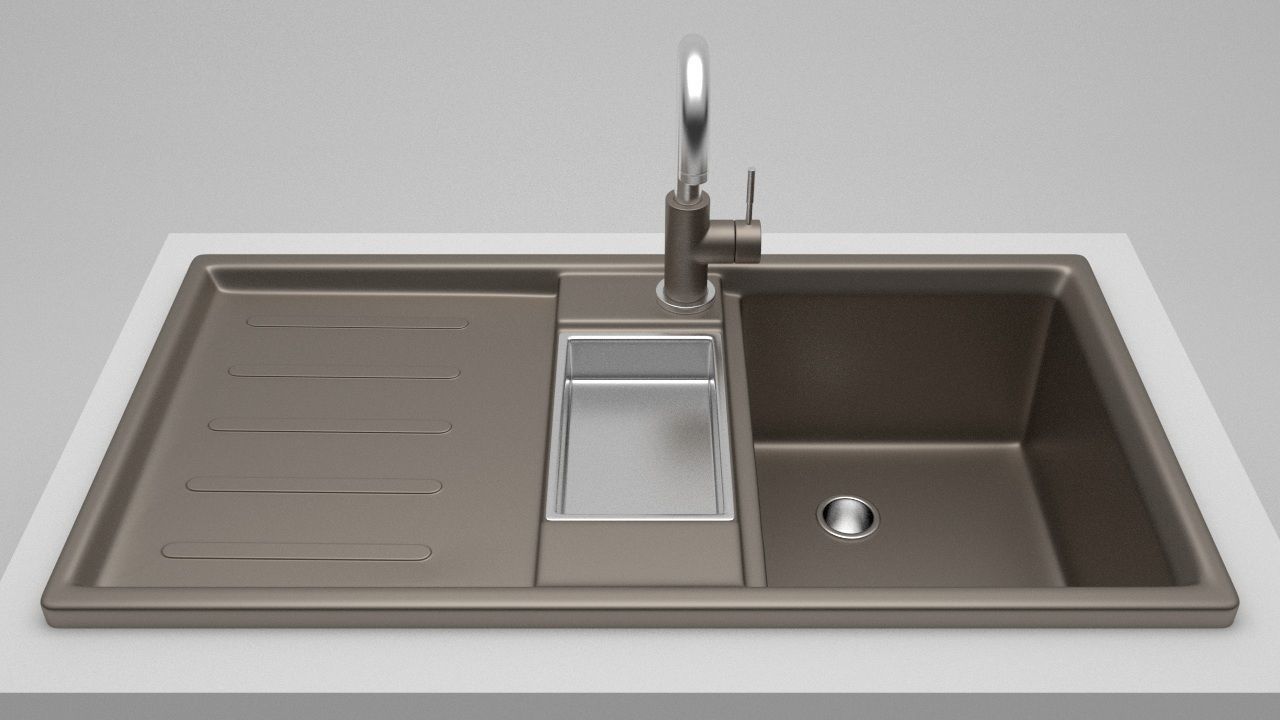
How the Kitchen Sink Model and Propensity Score Work Together
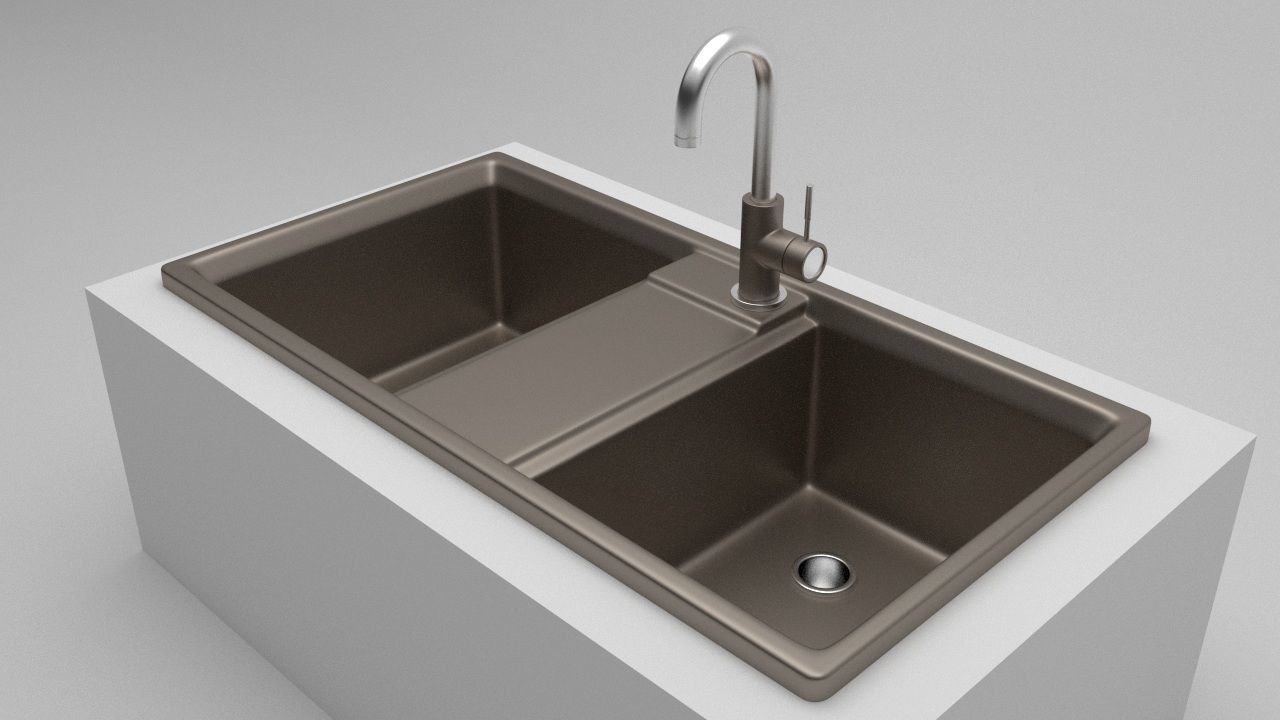
The Benefits of Using the Kitchen Sink Model Propensity Score

In Conclusion
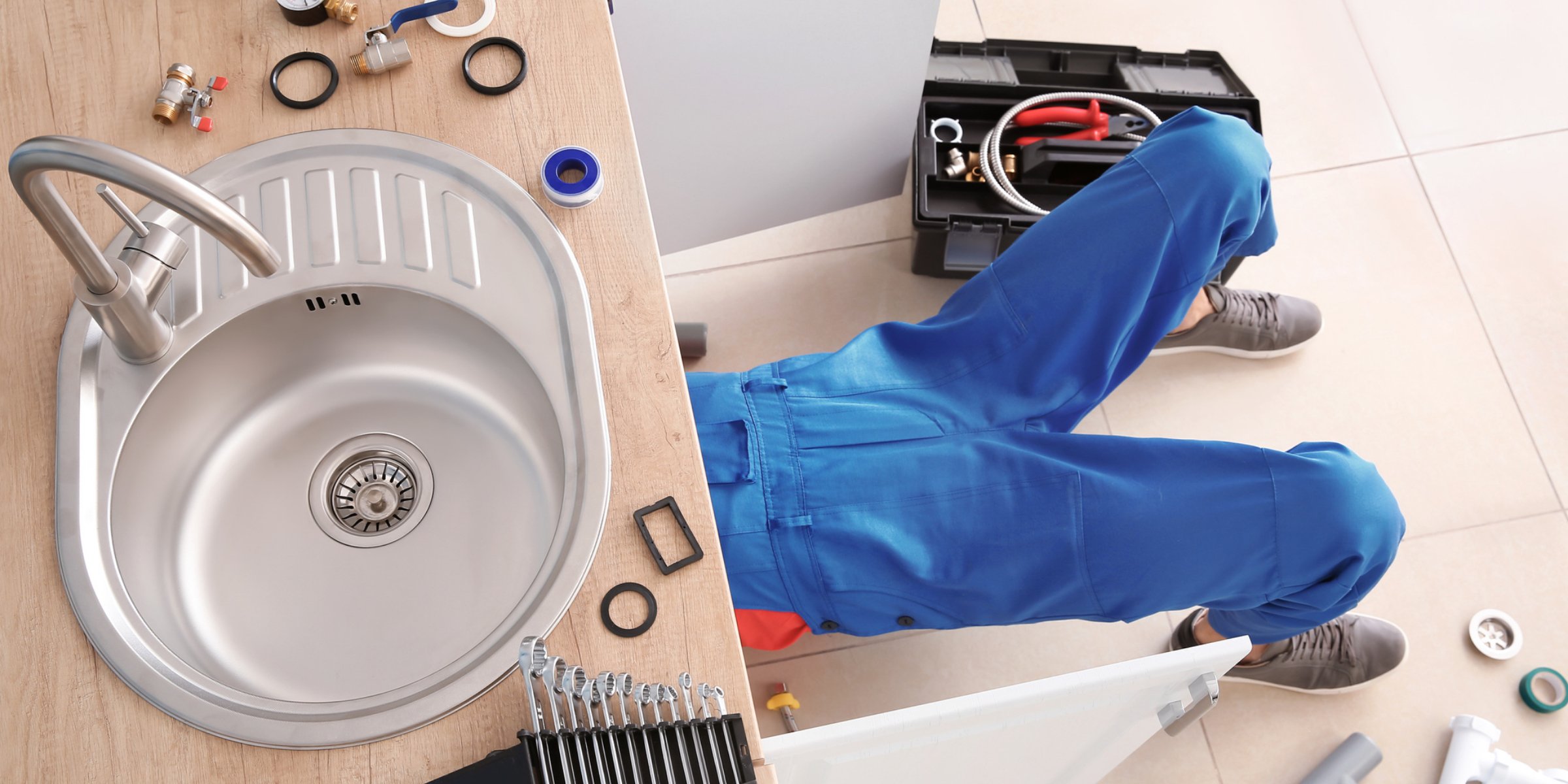