Linear regression is a commonly used statistical method for predicting a continuous outcome variable based on one or more independent variables. In R, linear regression can be performed using the lm() function. This function allows you to specify the formula for the regression model, as well as any additional arguments such as data or weights. The summary() function can be used to view the results of the regression, including the coefficients, p-values, and R-squared value.Linear Regression in R
Multiple linear regression is an extension of linear regression that allows for the inclusion of multiple independent variables in the model. In R, multiple linear regression can be performed using the lm() function, with the formula specifying all of the independent variables to be included. The summary() function can then be used to view the results, including the coefficients and p-values for each variable.Multiple Linear Regression in R
Logistic regression is a statistical method used for predicting a binary outcome variable (i.e. yes or no, success or failure) based on one or more independent variables. In R, logistic regression can be performed using the glm() function, with the family argument set to "binomial". The summary() function can then be used to view the results, including the coefficients and p-values for each variable.Logistic Regression in R
Poisson regression is a type of generalized linear model used for predicting count data. In R, Poisson regression can be performed using the glm() function, with the family argument set to "poisson". The summary() function can then be used to view the results, including the coefficients and p-values for each variable. Note that the dependent variable should be integer-valued for Poisson regression to be appropriate.Poisson Regression in R
Generalized linear models (GLMs) are a class of models that extend linear regression to allow for different types of response variables, such as binary or count data. In R, GLMs can be specified using the glm() function, with the family argument specifying the appropriate distribution for the response variable. The summary() function can then be used to view the results, including the coefficients and p-values for each variable.Generalized Linear Models in R
Stepwise regression is a method of selecting the most important variables to include in a regression model. In R, stepwise regression can be performed using the step() function, which automatically selects the best model based on a specified criterion such as AIC or BIC. This function can be used for both linear and generalized linear models.Stepwise Regression in R
Ridge regression is a technique used for dealing with multicollinearity (high correlations between independent variables) in linear regression models. In R, ridge regression can be performed using the glmnet() function from the glmnet package. This function allows you to specify the value of the tuning parameter, which determines the amount of shrinkage applied to the coefficients.Ridge Regression in R
Lasso regression is another technique for dealing with multicollinearity in linear regression models. In R, lasso regression can also be performed using the glmnet() function, with the family argument set to "gaussian" for continuous variables or "binomial" for binary variables. The tuning parameter can be specified using the lambda argument.Lasso Regression in R
Elastic net regression is a combination of ridge and lasso regression, allowing for both shrinkage and variable selection in a linear regression model. In R, elastic net regression can be performed using the glmnet() function, with the alpha argument determining the balance between ridge and lasso penalties. A value of 1 corresponds to lasso regression, while 0 corresponds to ridge regression.Elastic Net Regression in R
Bayesian regression is an alternative approach to traditional regression methods, using Bayesian statistics to estimate the model parameters. In R, Bayesian regression can be performed using the stan_glm() function from the rstanarm package. This function allows you to specify the prior distribution for the model parameters, as well as the number of iterations for the Markov chain Monte Carlo (MCMC) algorithm used for estimation.Bayesian Regression in R
The Importance of Kitchen Sink Regression in R for Effective House Design
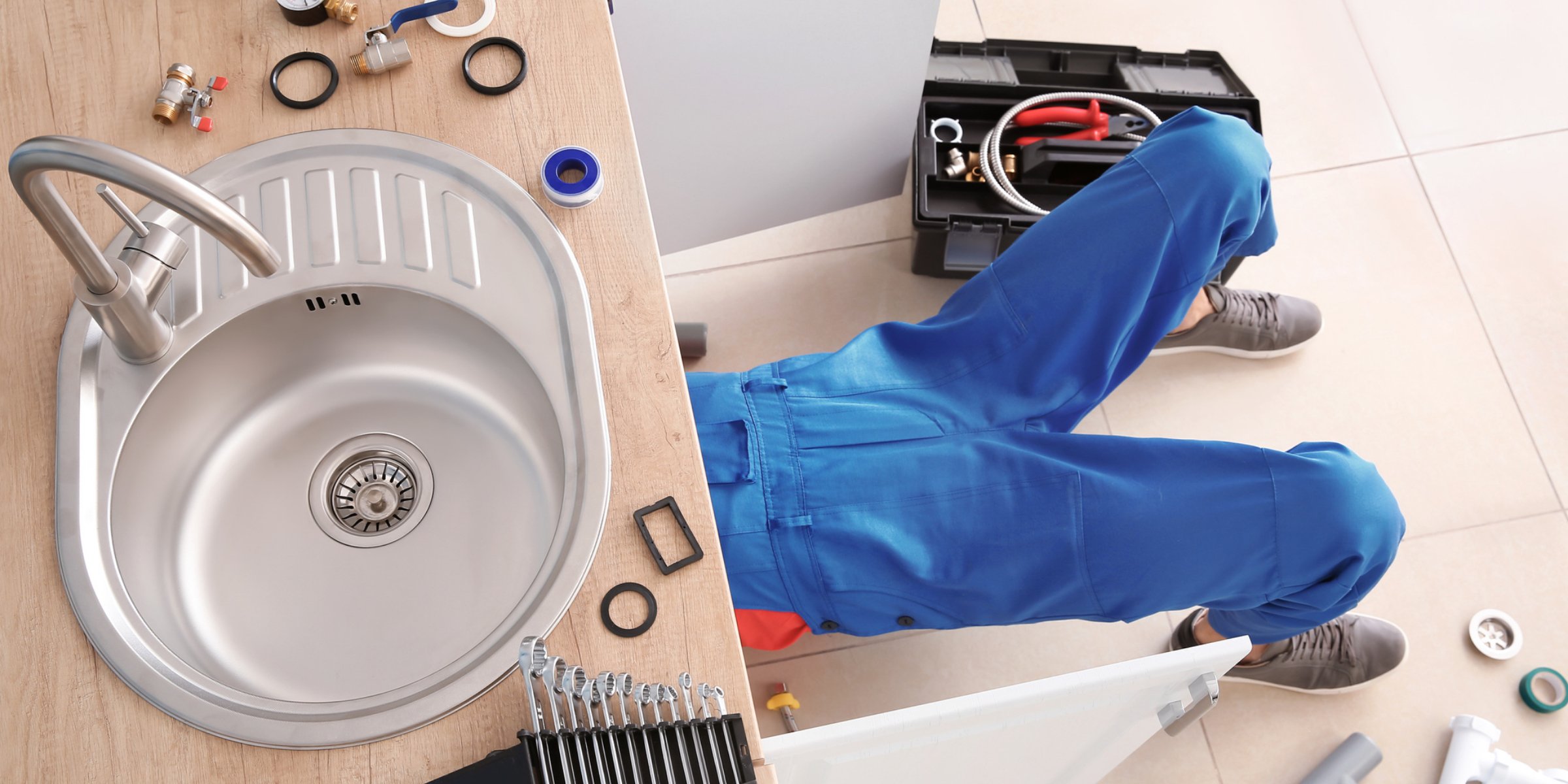
The Basics of Kitchen Sink Regression
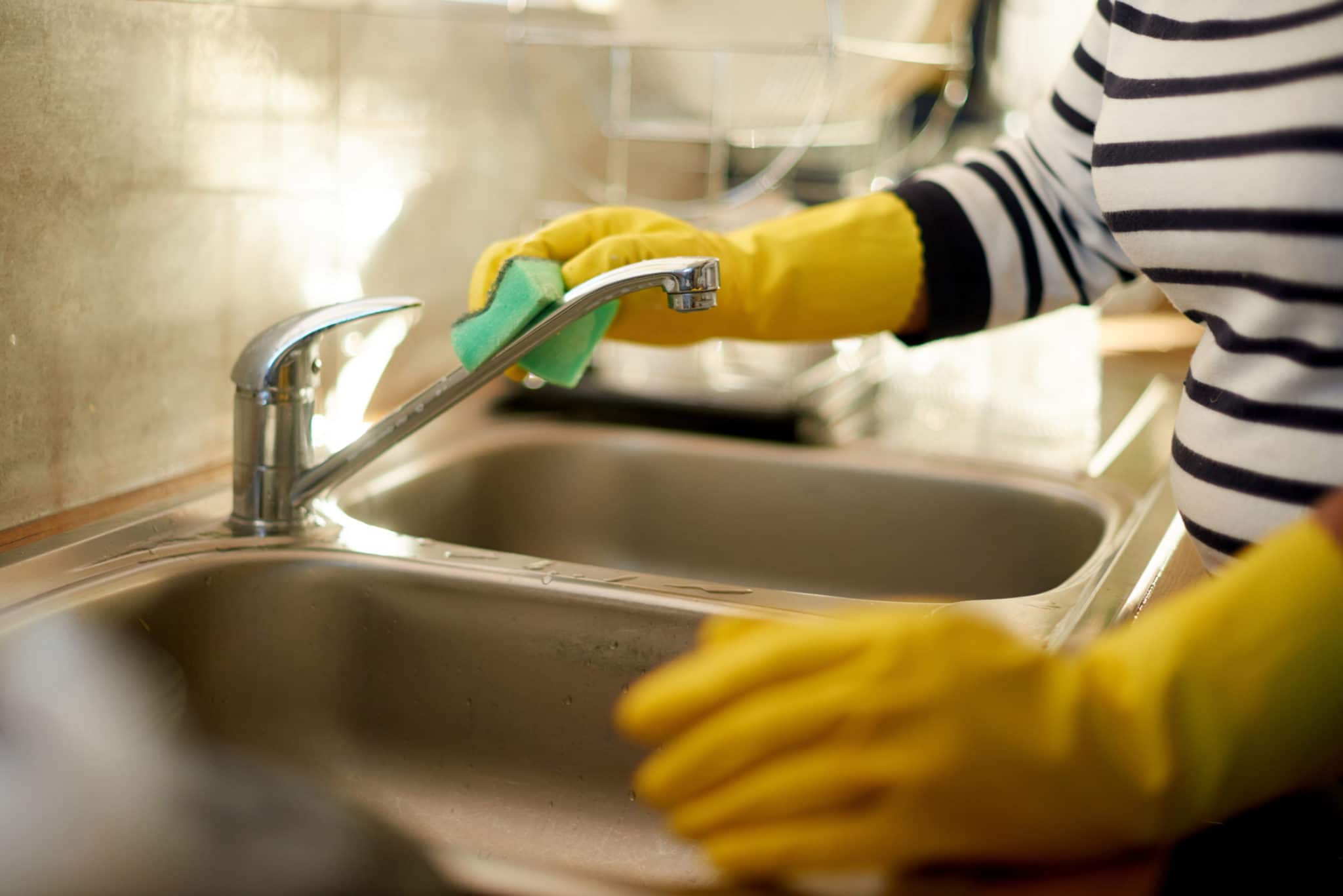
Why It Matters for House Design

Optimizing the Design Process
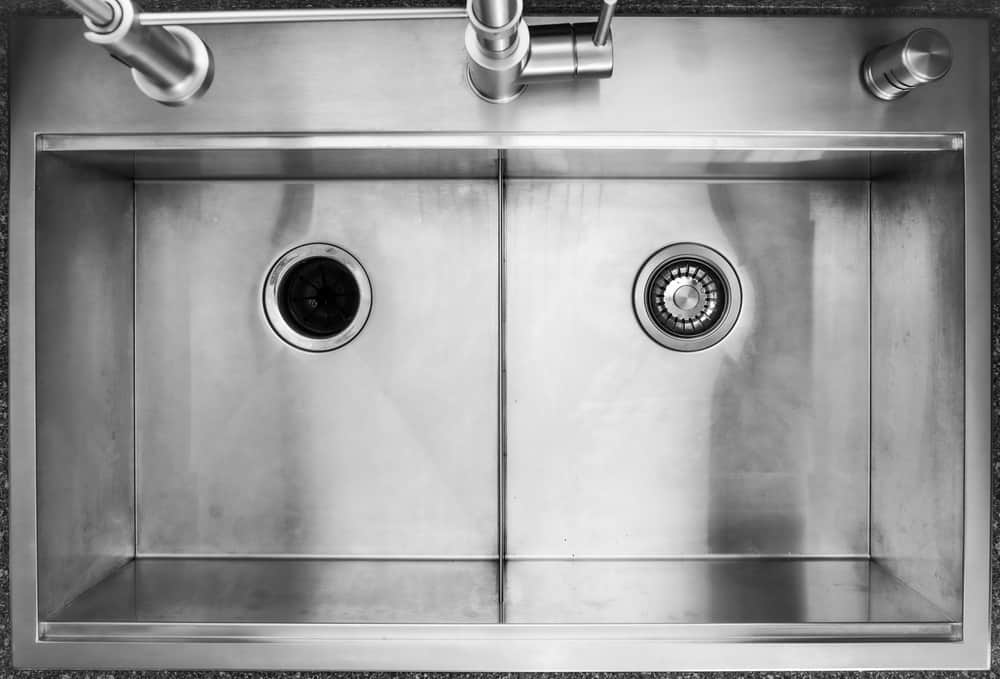
Future Applications in House Design
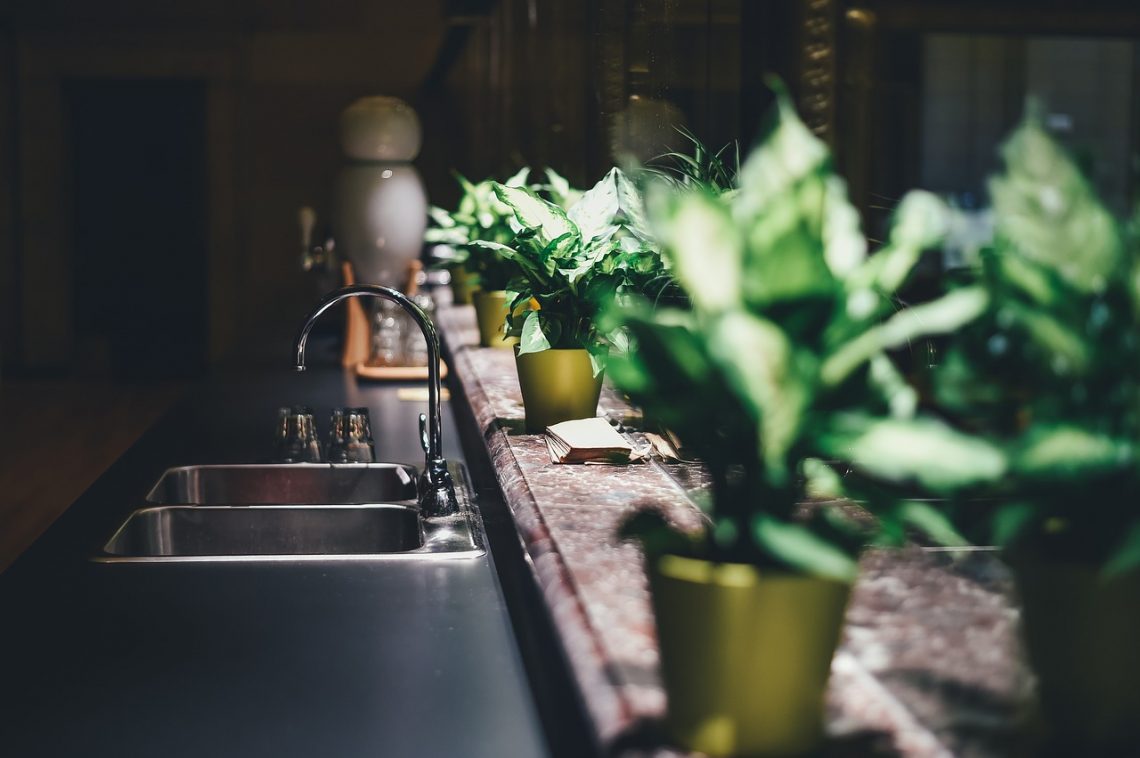