Linear regression is a statistical technique used to model the relationship between a dependent variable and one or more independent variables. In the world of regression analysis, the term "kitchen sink" refers to a model that includes every possible independent variable, regardless of its significance or relevance to the dependent variable. In this article, we will explore the concept of kitchen sink regression and provide examples of how it can be applied in the context of a kitchen sink model for predicting kitchen sink price.Linear Regression: Kitchen Sink Example
R is a popular programming language and environment for statistical computing and graphics. It is widely used by data scientists and analysts for data manipulation, visualization, and regression analysis. In R, the kitchen sink regression can be implemented by including all available independent variables in the model formula. However, it is important to note that this approach may result in overfitting and should be used with caution.Kitchen Sink Regression in R
Multiple linear regression is a statistical method that allows for the prediction of a continuous dependent variable using multiple independent variables. In the kitchen sink regression context, this means including all available independent variables in the model, regardless of their significance or correlation with the dependent variable. Let's consider an example where we want to predict the price of a kitchen sink using variables such as sink material, size, style, and brand.Multiple Linear Regression Example
Python is a popular language for data analysis and machine learning. It offers various libraries and packages for regression analysis, including the widely used scikit-learn library. In Python, the kitchen sink regression can be implemented by including all available independent variables in the model formula. However, as with R, it is important to be mindful of potential overfitting and to evaluate the model's performance.Kitchen Sink Regression in Python
Regression analysis is a statistical technique used to examine the relationship between a dependent variable and one or more independent variables. In a kitchen sink model, all available independent variables are included in the model, regardless of their significance or relevance to the dependent variable. This approach can be useful for identifying potential predictors and understanding their individual effects on the dependent variable.Regression Analysis: Kitchen Sink Model
SAS is a popular statistical software used for data analysis and predictive modeling. In SAS, the kitchen sink regression can be implemented using the "proc reg" procedure, where all available independent variables are included in the model formula. As with other programming languages, caution should be taken to avoid overfitting and to assess the model's performance.Kitchen Sink Regression in SAS
Stata is a statistical software package used for data analysis and visualization. In Stata, the kitchen sink regression can be implemented using the "regress" command with all available independent variables included in the model. As with other statistical software, it is important to evaluate the model's performance and consider potential overfitting.Kitchen Sink Regression in Stata
SPSS is a popular statistical software used for data analysis and predictive modeling. In SPSS, the kitchen sink regression can be implemented using the "regression" command with all available independent variables included in the model. As with other software, it is important to evaluate the model's performance and consider potential overfitting.Kitchen Sink Regression in SPSS
Interpreting a kitchen sink regression model can be challenging, as it includes all available independent variables, regardless of their significance or relevance to the dependent variable. In this case, it is essential to carefully evaluate the coefficients and their associated p-values to determine which variables are truly significant predictors and which may be the result of chance or overfitting.Kitchen Sink Regression Interpretation
As with any regression analysis, there are several assumptions that should be met for a kitchen sink regression model to be valid. These include linearity, normality, homoscedasticity, and independence of observations. Violations of these assumptions can lead to biased estimates and inaccurate predictions, so it is important to assess and address them before interpreting the results of a kitchen sink regression model.Kitchen Sink Regression Assumptions
The Importance of Kitchen Sink Regression in House Design

Understanding Kitchen Sink Regression
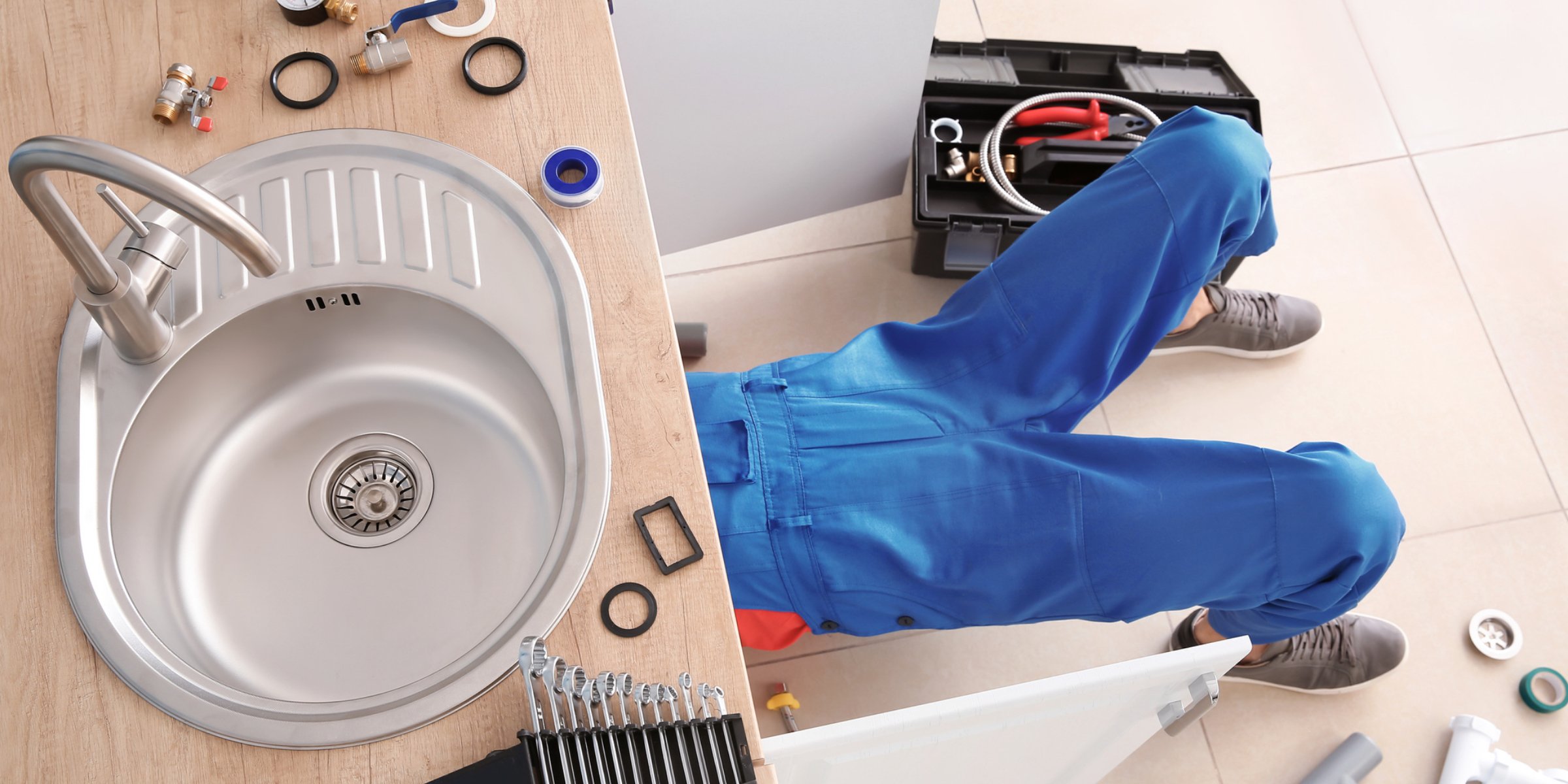
The Role of Kitchen Sink in House Design

Benefits of Kitchen Sink Regression
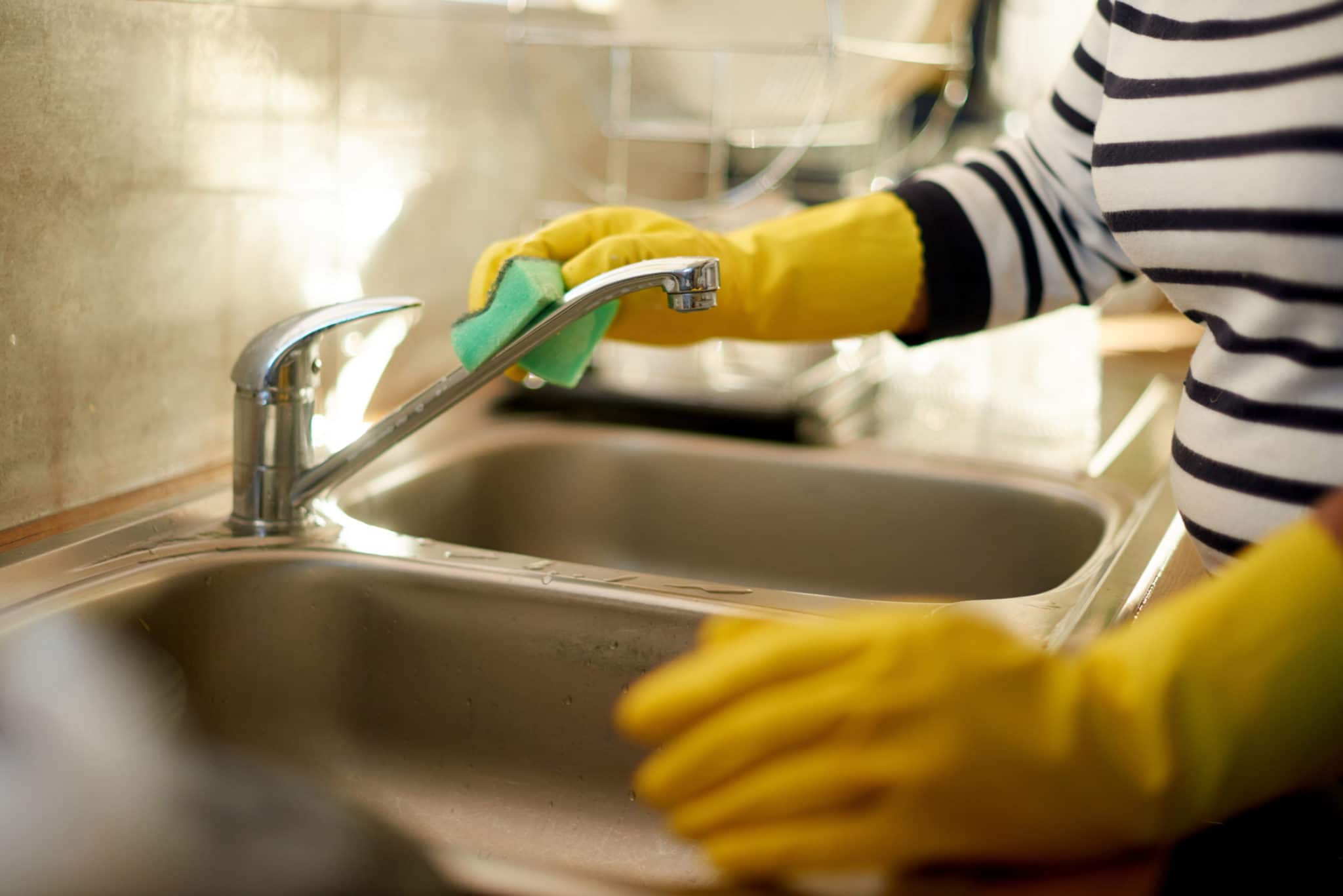
Examples of Kitchen Sink Regression
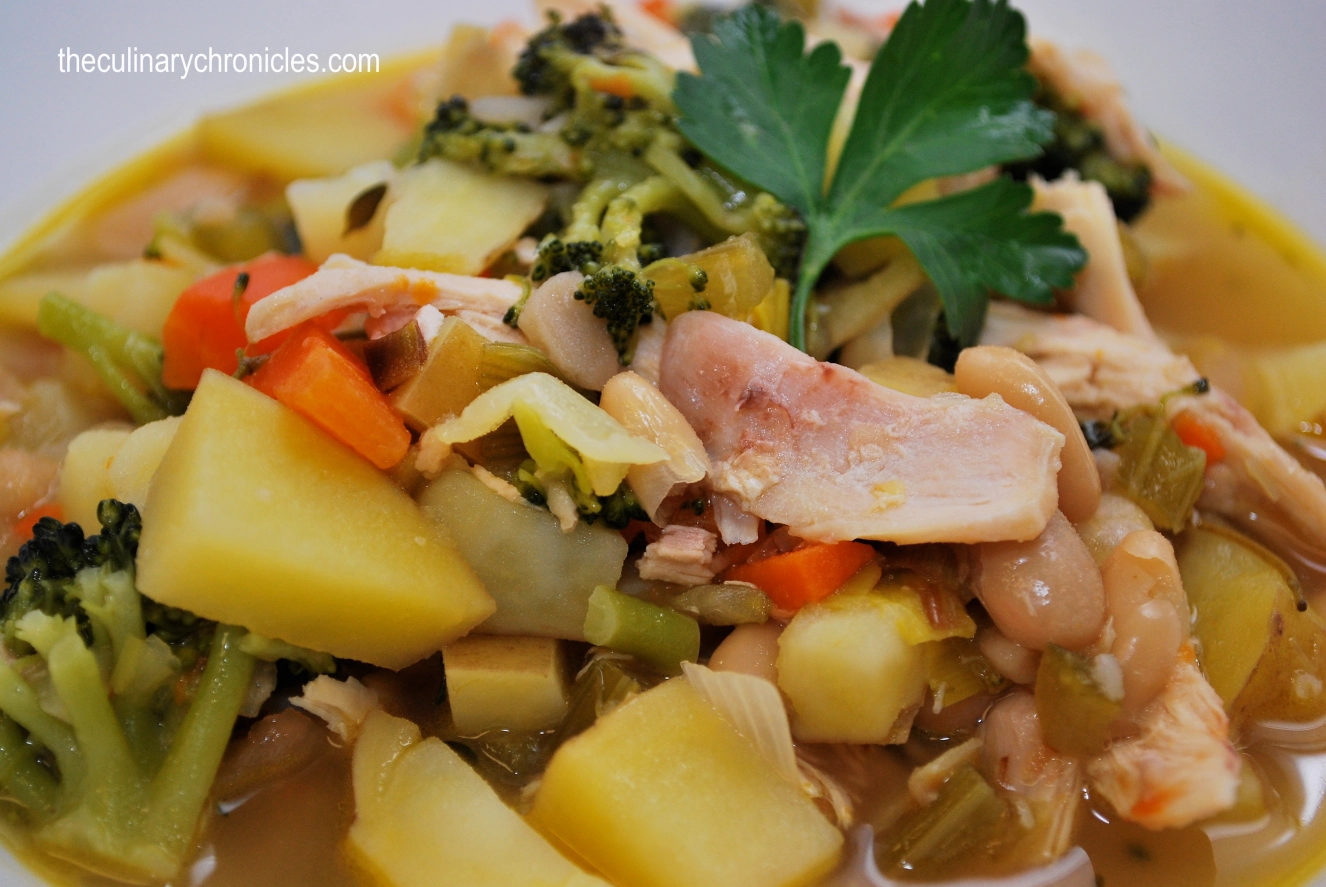
Conclusion
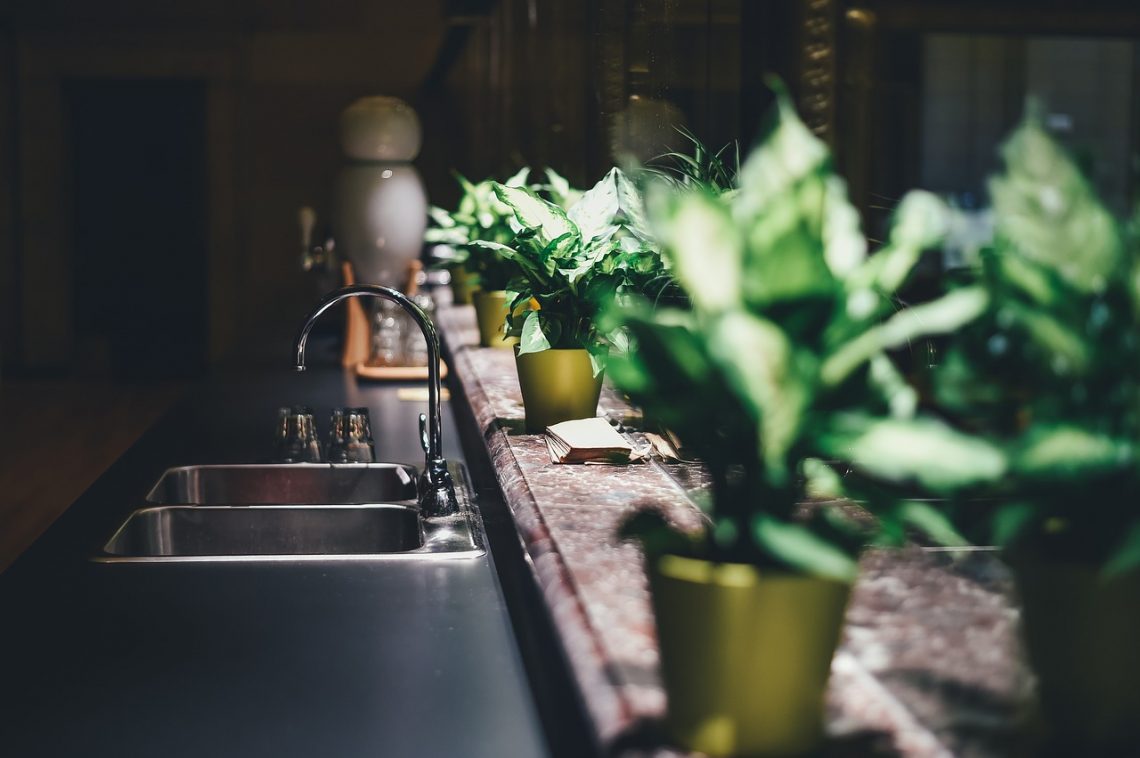