Kitchen Sink Regression is a statistical approach used in data analysis to predict the relationship between a dependent variable and multiple independent variables. It is called "kitchen sink" because it involves throwing in all possible variables, even those that may not have a significant impact on the outcome, in order to get a more comprehensive understanding of the data.What is Kitchen Sink Regression?
The first step in using the Kitchen Sink Regression approach is to gather all the possible variables that may have an impact on the dependent variable. This can include both quantitative and qualitative variables. Once all the variables are identified, they are then included in the regression model to see how they individually and collectively affect the outcome. The next step is to run the regression model and analyze the results. This will provide information on which variables have a significant impact on the dependent variable and which do not. The variables that do not have a significant impact can then be removed from the model to simplify it and make it more accurate.How to Use the Kitchen Sink Regression Approach
One of the main advantages of using the Kitchen Sink Regression approach is that it allows for a more comprehensive understanding of the data. By including all possible variables, it takes into account any potential factors that may affect the outcome. However, this approach also has its disadvantages. One of the main drawbacks is that including too many variables can lead to overfitting, where the model is too complex and only works well on the specific data it was trained on. This can result in poor performance when applied to new data.Advantages and Disadvantages of Kitchen Sink Regression
Kitchen Sink Regression is commonly used in fields such as economics, finance, and marketing. For example, in economics, it can be used to predict the impact of various economic factors on the stock market. In finance, it can be used to understand the relationship between different financial variables and a company's profitability. In marketing, it can be used to determine the factors that influence a customer's purchase decision.Examples of Kitchen Sink Regression in Practice
When interpreting the results from a Kitchen Sink Regression, it is important to look at the p-values for each variable. A p-value less than 0.05 indicates that the variable has a significant impact on the outcome. Additionally, the coefficient for each variable can also provide valuable information. A positive coefficient indicates a positive relationship between the variable and the outcome, while a negative coefficient indicates a negative relationship.How to Interpret Results from Kitchen Sink Regression
One common pitfall of Kitchen Sink Regression is including too many variables in the model, which can lead to overfitting as mentioned earlier. Additionally, it is important to ensure that the variables are not highly correlated with each other, as this can also affect the accuracy of the results. It is also important to carefully choose which variables to include in the model, as irrelevant or insignificant variables can also affect the results.Common Pitfalls of Kitchen Sink Regression
There are several alternative approaches to Kitchen Sink Regression, depending on the specific goals and data. These include stepwise regression, ridge regression, and lasso regression. Each of these approaches has its own strengths and weaknesses, and it is important to carefully consider which one is most suitable for the specific analysis.Alternatives to Kitchen Sink Regression
Choosing the right variables for Kitchen Sink Regression is crucial for obtaining accurate results. It is important to consider the theoretical basis for including each variable, as well as their statistical significance. Additionally, conducting thorough exploratory data analysis can also help identify potential variables that may have an impact on the outcome.How to Choose the Right Variables for Kitchen Sink Regression
Like any regression model, Kitchen Sink Regression is based on certain assumptions. These include the linearity of the relationship between the variables and the outcome, the normality of the residuals, and the absence of multicollinearity. It is important to check these assumptions before interpreting the results and making any conclusions from the model.Understanding the Assumptions of Kitchen Sink Regression
Kitchen Sink Regression can be implemented in various statistical software, including R and Python. In R, the "lm" function can be used to run a linear regression model, and in Python, the "statsmodels" library provides tools for regression analysis. It is important to have a strong understanding of the software and its functions before attempting to implement Kitchen Sink Regression.How to Implement Kitchen Sink Regression in R or Python
The Kitchen Sink Regression Approach: A Comprehensive Guide to House Design
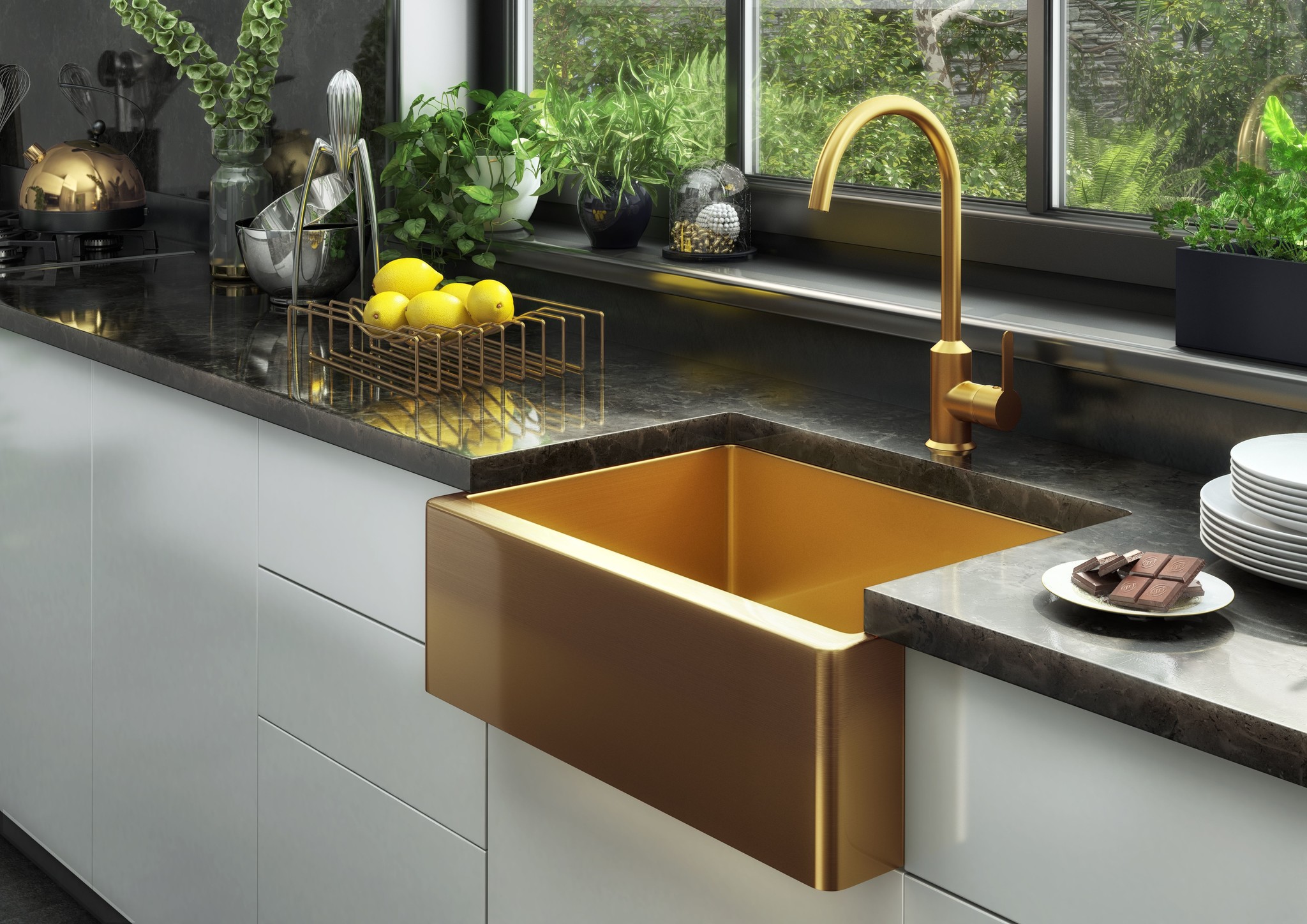
The Importance of a Well-Designed Kitchen

The All-Inclusive Approach
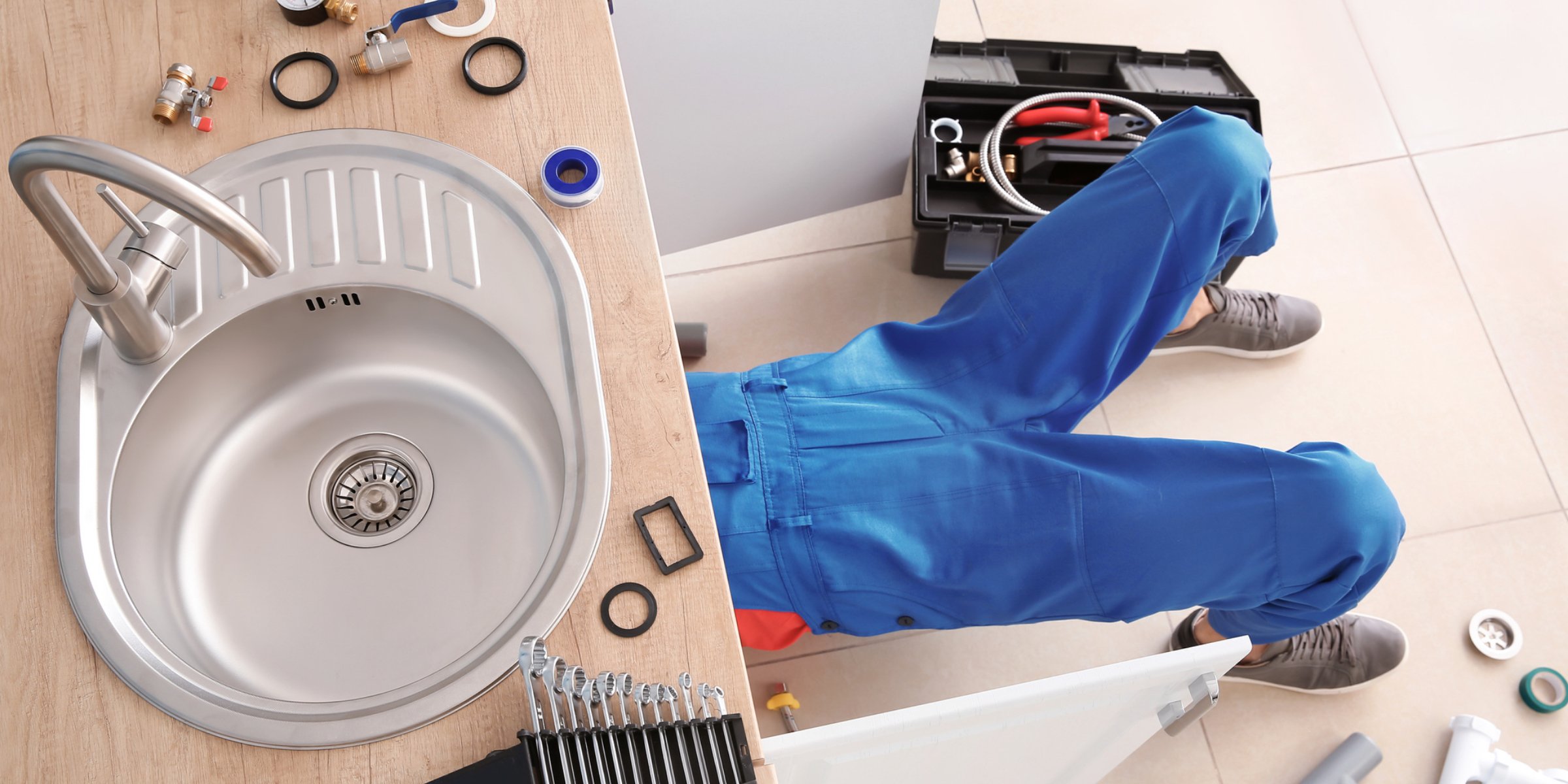
The Role of Personalization

Conclusion
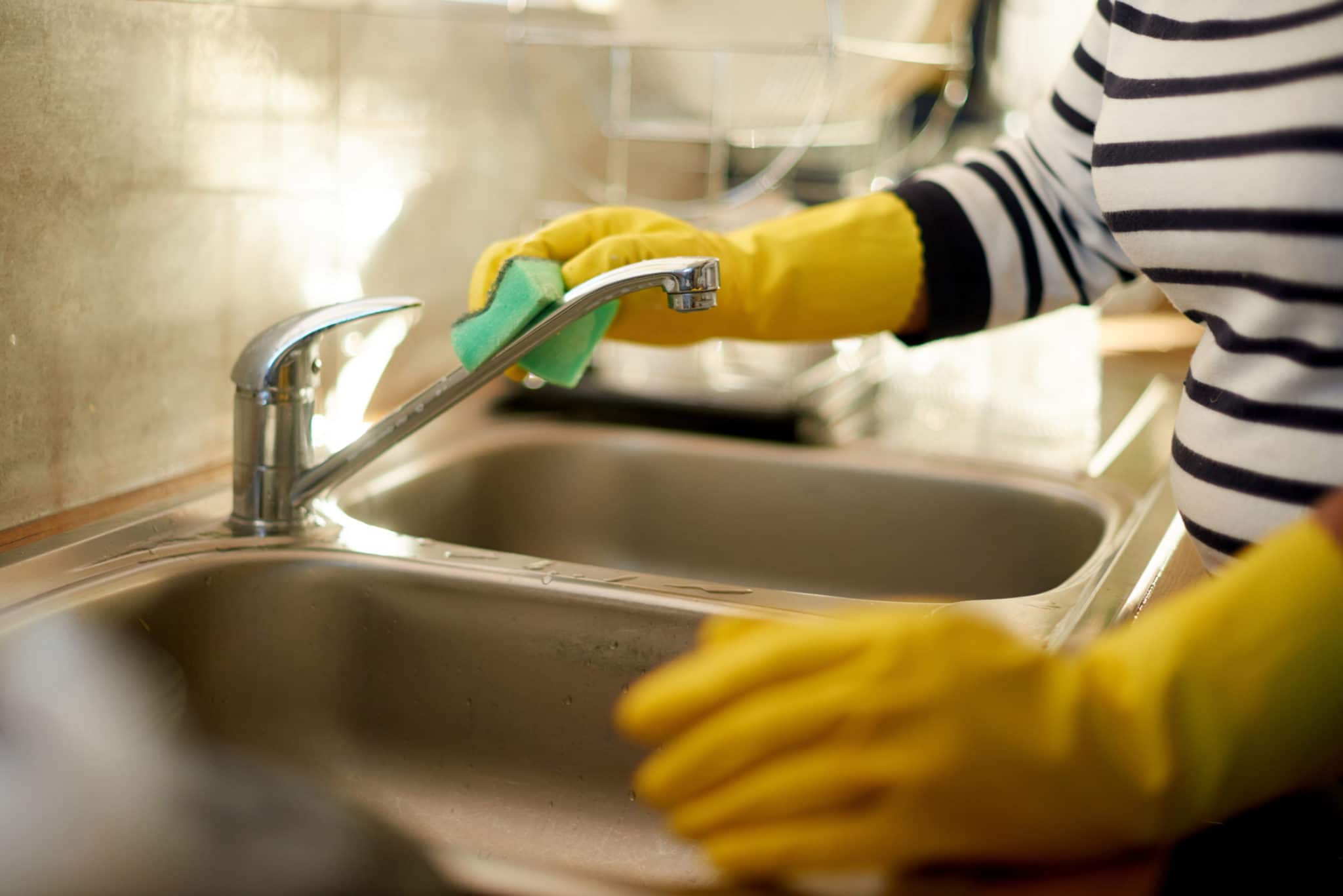